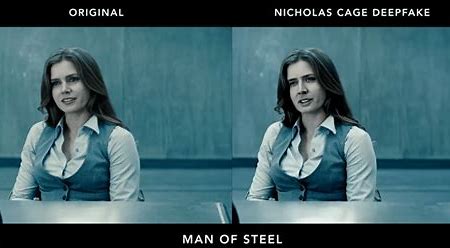
Deepfake video methods can digitally alter a person’s lip movements to match words that they never said. As part of an effort to grow awareness about such technologies through art, the MIT Center for Advanced Virtuality created a fake video showing former US President Richard Nixon giving a speech about astronauts being stranded on the moon.
Thus the widespread concern about deepfake technology, which has triggered an urgent search for answers among journalists, police investigators, insurance companies, human-rights activists, intelligence analysts and just about anyone else who relies on audiovisual evidence.
Among the leaders in that search has been Sam Gregory, a documentary filmmaker who has spent two decades working for Witness, a human-rights organisation based in Brooklyn, New York. One of Witness’ major goals, says Gregory, is to help people in troubled parts of the world take advantage of dramatically improved cell-phone cameras “to document their realities in ways that are trustworthy and compelling and safe to share.”
Unfortunately, he adds, “you can’t do that in this day and age without thinking about the downsides” of those technologies – deepfakes being a prime example. So in June 2018, Witness partnered with First Draft, a global non-profit that supports journalists grappling with media manipulation, to host one of the first serious workshops on the subject.
Technologists, human-rights activists, journalists and people from social-media platforms developed a roadmap to prepare for a world of deepfakes.
More Witness-sponsored meetings have followed, refining that roadmap down to a few key issues. One is a technical challenge for researchers: Find a quick and easy way to tell trustworthy media from fake. Another is a legal and economic challenge for big social media platforms such as Facebook and YouTube: Where does your responsibility lie? After all, says Hany Farid, “if we did not have a delivery mechanism for deepfakes in the form of social media, this would not be a threat that we are concerned about.”
And for everyone there is the challenge of education – helping people understand what deepfake technology is, and what it can do.
Farid is a computer scientist at the University of California, Berkeley, and author of an overview of image forensics in the 2019 Annual Review of Vision Science.
Deepfakes have their roots in the triumph of the “neural networks,” a once-underdog form of artificial intelligence that has re-emerged to power today’s revolution in driverless cars, speech and image recognition and a host of other applications.
Although the neural-network idea can be traced back to the 1940s, it began to take hold only in the 1960s, when AI was barely a decade old and progress was frustratingly slow. Trivial-seeming aspects of intelligence, such as recognising a face or understanding a spoken word, were proving to be far tougher to programme than supposedly hard skills like playing chess or solving cryptograms.
In response, a cadre of rebel researchers declared that AI should give up trying to generate intelligent behaviour with high-level algorithms – then the mainstream approach – and instead do so from the bottom up by simulating the brain.
To recognise what’s in an image, for example, a neural network would pipe the raw pixels into a network of simulated nodes, which were highly simplified analogues of brain cells known as neurons. These signals would then flow from node to node along connections: analogues of the synaptic junctions that pass nerve impulses from one neuron to the next.
Depending on how the connections were organised, the signals would combine and split as they went, until eventually they would activate one of a series of output nodes. Each output, in turn, would correspond to a high-level classification of the image’s content – “puppy,” for example, or “eagle,” or “George.”
The payoff, advocates argued, was that neural networks could be much better than standard, algorithmic AI at dealing with real-world inputs, which tend to be full of noise, distortion and ambiguity. (Say “service station” out loud. They’re two words – but it’s hard to hear the boundary.)
And better still, the networks wouldn’t need to be programmed, just trained. Simply show your network a few zillion examples of, say, puppies and not-puppies, like so many flash cards, and ask it to guess what each image shows. Then feed any wrong answers back through all those connections, tweaking each one to amplify or dampen the signals in a way that produces a better outcome next time.
The earliest attempts to implement neural networks weren’t terribly impressive, which explains the underdog status. But in the 1980s, researchers greatly improved the performance of networks by organising the nodes into a series of layers, which were roughly analogous to different processing centres in the brain’s cortex.
So, in the image example, pixel data would flow into an input layer; then be combined and fed into a second layer that contained nodes responding to simple features like lines and curves; then into a third layer that had nodes responding to more complex shapes such as noses; and so on.
Later, in the mid-2000s, exponential advances in computer power allowed advocates to develop networks that were far “deeper” than before – meaning they could be built not just with one or two layers, but dozens. The performance gains were spectacular.
In 2009, neural network pioneer Geoffrey Hinton and two of his graduate students at the University of Toronto demonstrated that such a “deep-learning” network could recognise speech much better than any other known method.
Then in 2012, Hinton and two other students showed that a deep-learning network could recognise images better than any standard vision system – and neural networks were underdogs no more. Tech giants such as Google, Microsoft and Amazon quickly started incorporating deep-learning techniques into every product they could, as did researchers in biomedicine, high-energy physics and many other fields.
The neural-network approach to artificial intelligence is designed to model the brain’s neurons and links with a web of simulated nodes and connections. Such a network processes signals by combining and recombining them as they flow from node to node.
Early networks were small and limited. But today’s versions are far more powerful, thanks to modern computers that can run networks both bigger and “deeper” than before, with their nodes organised into many more layers.
Yet as spectacular as deep learning’s successes were, they almost always boiled down to some form of recognition or classification – for example, Does this image from the drone footage show a rocket launcher? It wasn’t until 2014 that a PhD student at the University of Montreal, Ian Goodfellow, showed how deep learning could be used to generate images in a practical way.
Goodfellow’s idea, dubbed the generative adversarial network (GAN), was to gradually improve an image’s quality through competition – an ever-escalating race in which two neural networks try to outwit each other. The process begins when a “generator” network tries to create a synthetic image that looks like it belongs to a particular set of images – say, a big collection of faces.
That initial attempt might be crude. The generator then passes its effort to a “discriminator” network that tries to see through the deception: Is the generator’s output fake, yes or no? The generator takes that feedback, tries to learn from its mistakes and adjusts its connections to do better on the next cycle. But so does the discriminator – on and on they go, cycle after cycle, until the generator’s output has improved to the point where the discriminator is baffled.
The images generated for that first GAN paper were low-resolution and not always convincing. But as Facebook’s AI chief Yann LeCun later put it, GANs were “the coolest idea in deep learning in the last 20 years.”
Researchers were soon jumping in with a multitude of variations on the idea. And along the way, says Farid, the quality of the generated imagery increased at an astonishing rate. “I don’t think I’ve ever seen a technology develop as fast,” he says.
- A Wired report