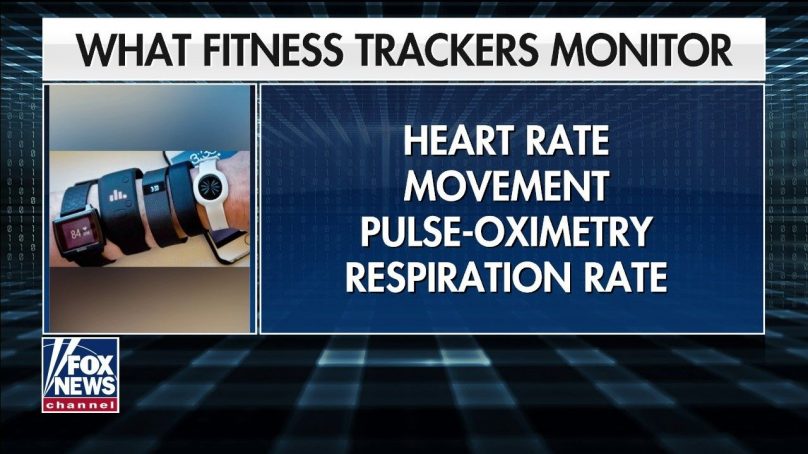
Five years ago, on a flight to Norway, Stanford University biologist Michael Snyder noticed that his body wasn’t behaving as it should. According to the multiple fitness trackers he happened to be wearing at the time, his heart rate was unusually high and his pulse ox – a measure of blood oxygen level – was unusually low.
“When I landed, they never came back to normal,” he says. “So, I knew something was up.”
Snyder could guess what that something was: Two weeks earlier, he’d helped his brother install a fence in rural Massachusetts – tick country. Sure enough, soon after landing in Norway, he developed a fever consistent with Lyme disease.
A Norwegian doctor gave him antibiotics to fight the infection until he returned home, when a test confirmed the diagnosis. “And the first clues were actually from my smartwatch and pulse ox,” Snyder says. “Pretty cool.”
Snyder was wearing the devices as part of an ongoing study, started in 2010, in which his lab is tracking wearable and other data from about a hundred people, including him. (As we speak, he flashes his wrists, brandishing no fewer than four smartwatches.)
“At the time we started, most people weren’t really even using them for health purposes,” he says – just to monitor daily activity.
“We realised, gosh, these are pretty good 24-7 monitors of your physiology.” He wondered what one could learn from all those data.
Maybe a lot. In a review of Snyder’s personal smartwatch data over the two years before his Lyme disease experience, his team found evidence for three viral infections that had already been confirmed by testing – including one that was asymptomatic.
“So, every time I was ill, we could pick it up with high heart rate and skin temperature – prior to symptom onset,” he says. The researchers began to design algorithms to identify deviations from baseline vitals in anyone, with the goal of combining genetic, wearable and other data to predict metabolic disorders, estimate cardiovascular risk and make other health assessments remotely.
Thus, began a research path – now joined by labs around the world – that could enable smartwatches to detect when people are infected with Covid-19 before they’re tested or even before they feel sick.
In recent years, Snyder and a number of other research groups have used wearable devices to monitor heart health and detect infectious diseases. Now, many have hope that the gadgets can be leveraged in the battle to stop the spread of Covid-19.
SARS-CoV-2, the virus that causes Covid-19, has infected more than 100 million people and killed more than two million. Accelerating its spread, people carrying the virus can transmit it to others without knowing they’re infected.
Massive rapid testing could curtail such transmission by alerting people to the infection, but most people don’t get tested every day and there wouldn’t be sufficient resources to do so anyway. Finding ways to quickly identify those most likely to test positive could save lives.
As Snyder suggests, the appeal of using smartwatches, fitness trackers and other such gadgets for this purpose is that they can monitor (depending on the device) heart rate, breathing rate, sleep, temperature, blood pressure and activity levels – and that tens of millions of Americans are already wearing them.
An illustration shows an idealised smartwatch and a few of its possible health-related functions, including the detection of heart rate, sleep activity, breathing intensity, temperature, physical activity and an alarm to warn a user of abnormal patterns.
Wearable devices can capture multiple vitals, including heart rate and activity levels and send alarms when something is off.
“We see a potential to help” with Covid-19, says Giorgio Quer, the director of artificial intelligence at Scripps Research Translational Institute and one of the leaders of DETECT, one of the largest efforts so far to test this idea.
In October, his team published a paper in Nature Medicine reporting on their findings in a study of 30,000 people who shared their health data last spring. They focused on device users who had been tested for Covid-19 at least once and who’d reported symptoms or lack thereof on a custom smartphone app.
The study used a common accuracy metric called AUC; a high AUC requires minimising both false positives and false negatives. The researchers’ primary question was whether wearable information – resting heart rate, sleep and activity – would add anything to self-reported symptoms. Indeed, it did.
Using only symptoms, the simple hand-coded algorithm scored an AUC of 0.71. Daily sensor data alone performed about the same — 0.72. But by adding sensors to symptoms, the AUC reached 0.80, a statistically significant improvement. “The findings there are really exciting,” Quer says.
In November, Snyder’s team at Stanford published a paper in Nature Biomedical Engineering describing their study of about 5,000 participants. It differs from the Scripps study in its resolution, zooming in on hour-by-hour changes in some measures.
The Stanford group’s algorithm collects data on three signals, all relative to the person’s baseline – a high resting heart rate (a result of inflammation), a high ratio of resting heart rate to daily steps taken and increased sleep (one way the body activates immune cells) – and looks for trends.
Among 32 device wearers who had experienced Covid-19 symptoms, it detected signals related to reported symptoms a median of four days before those symptoms appeared.
One limitation, though, is that this analysis, like the one at Scripps, was retrospective. That is, it looked back at data collected both before and after a prediction point, which is not of much use if you want to catch infection as it happens.
The eventual goal is a prospective system that detects possible illness in real time, helping wearers decide whether to seek testing or self-isolate.
Chart shows averaged changes in three variables detected by Fitbit devices on users who developed Covid-19. Breathing rate, heart rate and heart rate variability all shifted immediately before symptoms started with breathing rate and heart rate rising dramatically and heart rate variability decreasing. Levels returned closer to normal 20 days after symptoms first appeared.
Snyder’s team did also evaluate their system in prospective mode. They ran a simulation: At any given point, if they ignored data they had collected after that point, could their system detect illness, even if there had been no reported symptoms?
Twenty-four Fitbit wearers who’d gotten sick had enough presymptomatic data to test this hypothesis. In 15 of them, the system caught the illness.
- A Nature magazine report/Matthew Hutsons